Table Of Content
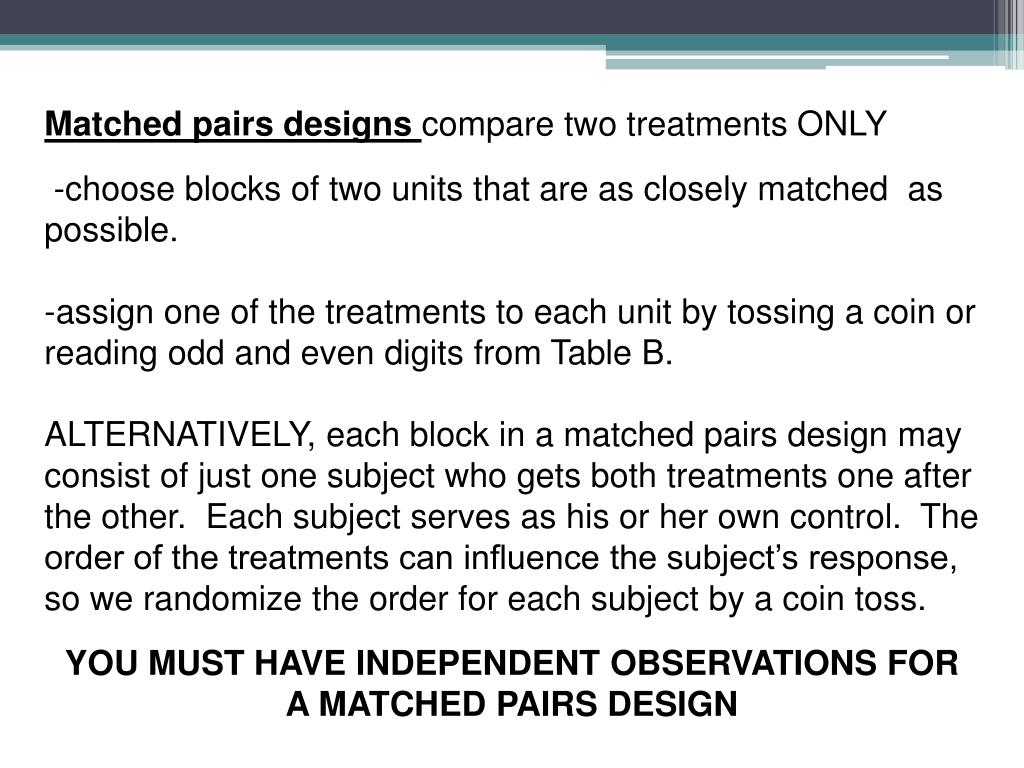
For quantitative data we are focused on means, while for categorical we are focused on proportions. In this chapter we will compare two means or two proportions to each other. The general procedure is still the same, just expanded. With two sample analysis it is good to know what the formulas look like and where they come from, however you will probably lean heavily on technology in preforming the calculations. The coach wants to know if the strength development class makes his players stronger, on average. Matching is especially useful in cases where participants can be paired with themselves.
Inference for Comparing Matched Pairs (HT for 2 Means, dependent samples)
In other words, we CANNOT explore alternative causal hypotheses since the design is definitive and cannot be changed. This will certainly be an issue since the causal association between risk factors, matching variables and outcome should be well understood in order to decide on which variable(s) to match. The calculator will do the subtraction, and you will have the differences in the third list.
15 Complementary Colors That Go with Brown - ELLE Decor
15 Complementary Colors That Go with Brown.
Posted: Tue, 03 Oct 2023 07:00:00 GMT [source]
Independent variable (IV)
I created this website to help researchers conduct studies from concept to publication. Keep in mind that, in general, we prefer to analyze the effect of variables that CAN be modified by people, such as smoking for example. Because once we prove the influence of these risk factors on the outcome, we can takes steps to help people modify their lifestyle in a certain direction.
MongoDB: How to Select a Random Sample of Documents
This similarity is crucial because it controls confounding variables, focusing solely on the treatment effects. In a Matched Pair Design, one subject from each pair receives one treatment, and the other subject receives another treatment. Researchers often use this design to tease apart the differences that the treatments may elicit. Delving into the realm of statistics, we often encounter experimental designs that aim to reduce variability and improve the reliability of results. It’s a tactic researchers employ to compare two treatments while minimizing differences between the subjects.
Matched pair design statistics offer a unique lens through which researchers can observe the effectiveness of interventions. This design reduces variability and increases the statistical power of the study. By looking at specific case studies, we can explore how this design enhances the reliability of results in various fields.
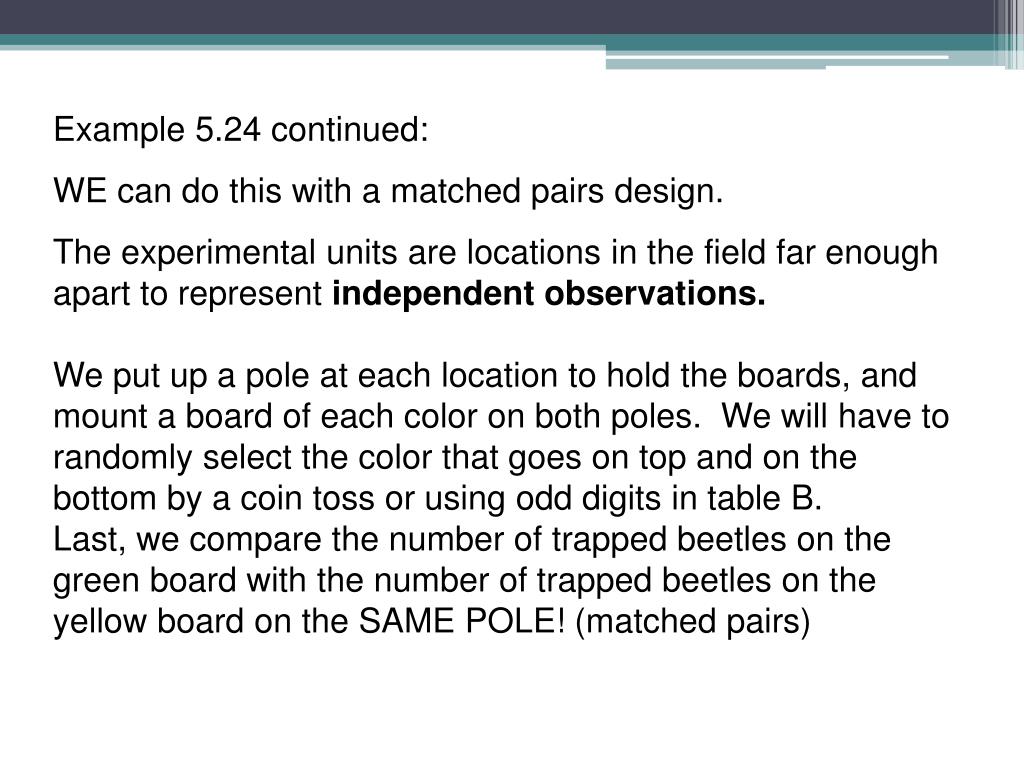
Experimental Design: Types, Examples & Methods
The groups are classified either as independent or dependent. Independent samples consist of two samples that have no relationship, that is, sample values selected from one population are not related in any way to sample values selected from the other population. Dependent samples consist of two groups that have some sort of identifiable relationship. Using the differences data, calculate the sample mean and the sample standard deviation. One member of each matched pair must be randomly assigned to the experimental group and the other to the control group. In cases where matching takes a lot of time and work to implement, we can instead invest in increasing the sample size and running a simple randomized controlled experiment.
16 Colors That Go With Red, According to Designers - Apartment Therapy
16 Colors That Go With Red, According to Designers.
Posted: Thu, 29 Feb 2024 08:00:00 GMT [source]
Repeated Measures design is also known as within-groups or within-subjects design. This should be done by random allocation, ensuring that each participant has an equal chance of being assigned to one group. For instance, in order to study the effect of a new sunscreen, the new product can be applied to the right arm (the treatment group), and the left arm can be used as control. On the off chance that one subject chooses to exit the review, you lose two subjects since you never again have a total pair. In our past model, each subject in the examination was just put on one eating regimen. I am Georges Choueiry, PharmD, MPH, and PhD student in epidemiology.
For instance, it probably won’t be difficult to come by 50 females to use as matches, yet it very well may be very elusive for 50 female matches in which each pair matches precisely on age. One of the major problems of matching is the difficulty to find appropriate matches. In some cases we may be forced to remove a number of participants from the study if appropriate matches could not be found.
It can be quite time-consuming to find subjects who match on certain variables, particularly if you use two or more variables. For example, it might not be hard to find 50 females to use as pairs, but it could be quite hard to find 50 female pairs in which each pair matches exactly on age. Matched pair experiments shine in statistical analysis. They allow researchers to compare two sets of data that are linked. But once the experiment ends, the real work begins—analyzing the data.
Therefore, lessening demand attributes might expand the legitimacy of the research. Order impact alludes to contrasts in results because of the order where trial materials are introduced to subjects. By utilizing a matched pair design, you don’t need to stress over order impact since each subject just gets one treatment. The obvious pro is that you can find matches more easily, but the con is that the subjects will match less precisely. For example, using the approach above it’s possible for a 21-year-old and a 25-year-old to be matched up, which is a rather notable difference in age. This is a trade-off that researchers must decide is worth or not in order to find pairs more easily.
Let’s explore this design’s essence and its advantages in research. A new prep class was designed to improve SAT test scores. Their scores on two practice exams were recorded, one before the class and one after. Understanding matched pair design statistics is crucial for precise data analysis. By recognizing the value of pairing subjects, researchers can significantly reduce variability.
For example, a lot of outcomes are gender and age specific. Therefore, matching individuals on these 2 variables will help improve the validity of the study by reducing bias. For example, maybe researchers are interested in the effect aspirin has in preventing heart attacks. One group is given aspirin and the other a placebo, and the heart attack rate is studied over several years.
The alternative hypothesis is negative, meaning there is less pain felt after hypnotism. The score should be lower after hypnotism, so the difference ought to be negative to indicate improvement. In this model we take the difference of each pair and create a new population of differences, so if effect, the hypothesis test is a one population test of mean that we already covered in the prior section. Condition one attempted to recall a list of words that were organized into meaningful categories; condition two attempted to recall the same words, randomly grouped on the page. To assess the effect of the organization on recall, a researcher randomly assigned student volunteers to two conditions.
No comments:
Post a Comment